Workshop : Approches topologiques et géométriques pour l’apprentissage statistique, théorie et pratique.
Le 28 septembre 2020, de 9h à 13h.
Présentation
Dans l’industrie, la multiplication des sources de données disponibles à l’analyse va souvent de pair avec une complexité croissante de ces données et leur appartenance à des espaces de grande dimension ou des espaces non euclidiens plus abstraits. Comprendre les structures topologiques et géométriques des données peut permettre de mieux les représenter puis les modéliser.
C’est l’objet de l’analyse topologique et géométrique des données. Ce workshop d’une demi-journée, co-organisé par SystemX et EDF, vise à réunir industriels et académiques autour de ce thème en pleine évolution. Les aspects théoriques et la mise en œuvre pratique de ces approches seront développés.
Lieu
Cet événement se tiendra à distance.
Inscriptions
Inscription préalable via ce lien.
Programme
9h30 – 10h30 : Frédéric Chazal – Directeur de recherche, Inria Saclay
Apprendre et exploiter la forme des données : une petite introduction à l’Analyse Topologique des Données.
L’Analyse Topologique des Données (TDA) est un domaine récent, à la croisée des mathématiques, de l’algorithmique et de la statistique, qui connait un succès croissant depuis quelques années. Il vise à comprendre, analyser et exploiter la structure topologique et géométrique de données complexes. Avec l’émergence de la théorie de la persistance topologique, la géométrie et la topologie algorithmique ont fourni des outils nouveaux et efficaces pour aborder ces questions. Dans cet exposé, nous mettrons en lumière quelques problématiques qui se posent lorsqu’on cherche à estimer des propriétés topologiques ou géométriques à partir de données et nous présenterons quelques approches et techniques fondamentales permettant de s’y attaquer.
Nous illustrerons aussi, sur quelques exemples issus d’applications concrètes, l’intérêt des approches topologiques pour l’analyse des données et l’apprentissage statistique.
Learning and exploiting the shape of data: a brief introduction to Topological Data Analysis.
Topological Data Analysis (TDA) is a recent and fast growing field whose aim is to analyze, understand and exploit the topological and geometric structure of data. With the emergence of the mathematical theory of persistent homology, computational topology and geometry have provided a set of new efficient and mathematically well-founded topological and geometric tools to achieve this goal. This talk is an introduction to a few fundamental approaches and methods, including persistent homology, to estimate relevant topological information about data and take advantage of it in further learning tasks.
We will illustrate the interest of topological approaches on a few examples coming from concrete applications.
Biography
Frederic Chazal is a Directeur de Recherche (senior researcher) at INRIA Saclay Ile-de-France since 2007. After a PhD in pure mathematics, he oriented his research to computational geometry and topology. He is now leading the DataShape team at INRIA, a group working on Topological Data Analysis (TDA), a recent fast growing field at the crossing of mathematics, statistics, machine learning and computer science.
Frederic’s contributions to the field go from fundamental mathematical aspects to algorithmic and applied problems. He published more than 80 papers in major computer sciences conferences and mathematics journals, he co-authored 2 reference books and 3 patents. He is, or has been, also an associate editor of 4 international journals: Discrete and Computational Geometry (Springer), SIAM Journal on Imaging Science, Graphical Models (Elsevier), Journal of Applied and Computational Topology (Springer).
During the last few years Frederic has been heading several national and international research projects on geometric and topological methods in statistics and machine learning. He is also the scientific head of joint industrial research projects between Inria and several companies such as Fujitsu (TDA, Machine Learning and explainable AI) or the French SME Sysnav.
________________________________________________________________________________________________________
10h30 – 11h30 : Frédéric Barbaresco – SENSING Segment Leader, Key Technology Domain PCC (Processing, Control & Cognition), THALES
Lie Group Machine Learning and Natural Gradient from Information Geometry: Souriau Lie Groups Thermodynamics, Koszul-Souriau-Fisher Metric and Entropy as Casimir Invariant Function
The classical simple gradient descent used in Deep Learning has two drawbacks: the use of the same non-adaptive learning rate for all parameter components, and a non-invariance with respect to parameter re-encoding inducing different learning rates. As the parameter space of multilayer networks forms a Riemannian space equipped with Fisher information metric, instead of the usual gradient descent method, the natural gradient or Riemannian gradient method, which takes account of the geometric structure of the Riemannian space, is more effective for learning. The natural gradient preserves this invariance to be insensitive to the characteristic scale of each parameter direction. The Fisher metric defines a Riemannian metric as the Hessian of two dual potential functions (the Entropy and the Massieu Characteristic Function).
In Souriau’s Lie groups thermodynamics, the invariance by re-parameterization in information geometry has been replaced by invariance with respect to the action of the group. In Souriau model, under the action of the group, the entropy and the Fisher metric are invariant. Souriau defined a Gibbs density that is covariant under the action of the group. The study of exponential densities invariant by a group goes back to the work of Muriel Casalis in her 1990 thesis. The general problem was solved for Lie groups by Jean-Marie Souriau in Geometric Mechanics in 1969, by defining a « Lie groups Thermodynamics » in Statistical Mechanics. These new tools are bedrocks for Lie Group Machine Learning. Souriau introduced a Riemannian metric, linked to a generalization of the Fisher metric for homogeneous Symplectic manifolds. This model considers the KKS 2-form (Kostant-Kirillov-Souriau) defined on the coadjoint orbits of the Lie group in the non-null cohomology case, with the introduction of a Symplectic cocycle, called « Souriau’s cocycle », characterizing the non-equivariance of the coadjoint action (action of the Lie group on the moment map).
Based on Souriau Lie groups Thermodynamics, we will prove that Entropy could be built as a generalized Casimir invariant function in coadjoint representation, and Massieu characteristic function, dual of Entropy by Legendre transform, as a generalized Casimir function in adjoint representation. This geometric structure is a foundation for a new geometric theory of information. The dual Lie algebra foliates into coadjoint orbits that are also the level sets on the entropy. The KKS 2-form, and the Souriau-Koszul-Fisher metric make each orbit into homogeneous Symplectic and kähler manifolds. The information manifold foliates into level sets of the entropy where motion remaining on this complex surfaces is non-dissipative, whereas motion transversal to these surfaces is dissipative.
We will introduce the link between Koszul geometry of homogeneous bounded domains, Souriau « Lie Groups Thermodynamics », Information Geometry and Kirillov representation theory to define probability densities as Souriau covariant Gibbs densities (density of Maximum of Entropy). We will illustrate this case for the matrix Lie group SU (1,1) (case with null cohomology), and the one for the matrix Lie group SE(3) (case with non-null cohomology), through the computation of Souriau’s moment map, and Kirillov’s orbit method.
Références
[1] Souriau, J.-M. : Structure des systèmes dynamiques, Dunod, (1969)
[2] Souriau, J.-M. : Mécanique statistique, groupes de Lie et cosmologie, Colloques int. du CNRS numéro 237. In Proceedings of the Géométrie Symplectique et Physique Mathématique, Aix-en-Provence, France, 24–28, pp. 59–113, (1974)
[3] Marle, C.-M. : From Tools in Symplectic and Poisson Geometry to J.-M. Souriau’s Theories of Statistical Mechanics and Thermodynamics. Entropy, 18, 370, (2016).
[4] Barbaresco, F. : Higher Order Geometric Theory of Information and Heat Based on Poly-Symplectic Geometry of Souriau Lie Groups Thermodynamics and Their Contextures: The Bedrock for Lie Group Machine Learning. Entropy, 20, 840, (2018)
[5] Barbaresco F.: Jean-Louis Koszul and the Elementary Structures of Information Geometry, SPRINGER Book Geometric Structure of Information, pp 333-392, SPRINGER, (2018)
[6] Casalis M.: Familles Exponentielles Naturelles Invariantes par un Groupe. Ph.D. Thesis, Thèse de l’Université Paul Sabatier, Toulouse, France, (1990)
[7] Tojo, K.; Yoshino, T. : A Method to Construct Exponential Families by Representation Theory. arXiv:1811.01394, (2018)
[8] Barbaresco F.: Lie Group Machine Learning and Gibbs Density on Poincaré Unit Disk from Souriau Lie Groups Thermodynamics and SU(1,1) Coadjoint Orbits. In: Nielsen, F., Barbaresco, F. (eds.) GSI 2019. LNCS, vol. 11712, SPRINGER, (2019)
[9] Barbaresco F.: Lie Group Statistics and Lie Group Machine Learning based on Souriau Lie Groups Thermodynamics & Koszul-Souriau-Fisher Metric: New Entropy Definition as Generalized Casimir Invariant Function in Coadjoint Representation, Submitted to MDPI Special Issue « Lie Group Machine Learning and Lie Group Structure Preserving Integrators », https://www.mdpi.com/journal/entropy/special_issues/Lie_group
[10] Koszul Jean-Louis : Introduction to Sympletic Geometry, SPRINGER 2019, https://link.springer.com/book/10.1007%2F978-981-13-3987-5
[11] Colloque « SOURIAU’19 »: http://souriau2019.fr/
[12] Colloque « FGSI’19 Cartan-Koszul Souriau – Foundation of Geometric Structures of Information »: https://fgsi2019.sciencesconf.org/
[13] Colloque « GSI’19 Geometric Science of Information »: https://www.see.asso.fr/GSI2019
[14] Les Houches Summer Week, Joint Structures and Common Foundations of Statistical Physics, Information Geometry and Inference for Learning (SP+IG’20), 26th July to 31st July 2020, https://franknielsen.github.io/SPIG-LesHouches2020/
Biography Frédéric Barbaresco received his State Engineering degree from the French Grand Ecole CentraleSupélec, Paris, France, in 1991. Since then, he has worked for the THALES Group where he is now SENSING Segment Leader of Key Technology Domain PCC (Processing, Control & Cognition). He has been an Emeritus Member of SEE since 2011 and he was awarded the Aymé Poirson Prize (for application of sciences to industry) by the French Academy of Sciences in 2014, the SEE Ampere Medal in 2007, the Thévenin Prize in 2014 and the NATO SET Lecture Award in 2012. He is President of SEE Technical Club ISIC “Engineering of Information and Communications Systems”. He was member of the administrative board of SMAI. He was an invited lecturer for UNESCO on “Advanced School and Workshop on Matrix Geometries and Applications” in Trieste at the ITCP in June 2013. He is the General Co-chairman of the new international conference GSI “Geometric Sciences of Information”. He was co-editor of MDPI Entropy Books “Information, Entropy and Their Geometric Structures” and « Joseph Fourier 250th Birthday: Modern Fourier Analysis and Fourier Heat Equation in Information Sciences for the XXIst century« . He has co-organized the CIRM seminar TGSI’17 “Topological and Geometrical Structures of Information”, “FGSI’19 Cartan-Koszul-Souriau” in 2019, and Les Houches Summer Week “Joint Structures and Common Foundations of Statistical Physics, Information Geometry and Inference for Learning” in 2020. He was keynote speaker at SOURIAU’19 event for 5Oth birthday of “structure des systèmes dynamiques”.
________________________________________________________________________________________________________
11h45 – 12h15 : Hatem Hajri and Hadi Zaatiti – Research engineers, IRT SystemX
Geometric methods for learning on manifolds and graphs: Theory and practice
This talk consists of two parts
1) We present “geomstats”, an open-source Python package for computations and statistics for data on non-linear manifolds such as hyperbolic spaces, spaces of symmetric positive definite matrices, Lie groups of transformations, etc. We provide object-oriented and extensively unit-tested implementations. The manifolds come with families of Riemannian metrics, with associated Exponential/Logarithm maps, geodesics, and parallel transport. The learning algorithms follow scikit-learn API and provide methods for estimation, clustering and dimension reduction on manifolds. The operations are vectorized for batch computations and available with NumPy, PyTorch, and TensorFlow backends, which allows GPU acceleration. We present the package, compare it with related libraries, and show relevant examples. Code and documentation: https://github.com/geomstats/geomstats
2) We present algorithms and packages to learn nodes and communities on graphs. These algorithms are developed based on the recent framework of hyperbolic embeddings and using Riemannian mixture models. We illustrate the performance of these methods by experiments on real-world social networks such as Wikipedia, Flickr, DBLP and BlogCatalog.
Biographies
Hatem Hajri is a senior research scientist at IRT SystemX, where he mainly works on robustness and adversarial attacks of artificial intelligence-based systems. Previously, he held three teaching and research positions as University Paris 10, Luxembourg University, and University of Bordeaux, where he worked on various problems of stochastic analysis and graphical models, and at the VeDeCoM Institute at Versailles, France, where he conducted research on autonomous driving. He earned the French agrégation of mathematics and his MS and PhD degrees in applied mathematics at Paris Sud University, France.
Hadi Zaatiti works as a research engineer at the Institute of Research and Technology IRT-SystemX on two aspects: system architecture of autonomous shuttles and learning graph data representations using Riemannian geometry. Hadi obtained an electrical engineering degree, a masters in system architecture at CentraleSupélec and a Ph.D in computer science from Université Paris-Saclay and the French Commissionary of Atomic Energy.
________________________________________________________________________________________________________
12h15 : Buffet déjeunatoire
Contacts
Yannig Goude : yannig.goude [at] edf.fr
Hatem Hajri : hatem.hajri [at] irt-systemx.fr
Georges Hebrail : georges.hebrail [at] edf.fr
Organisateurs
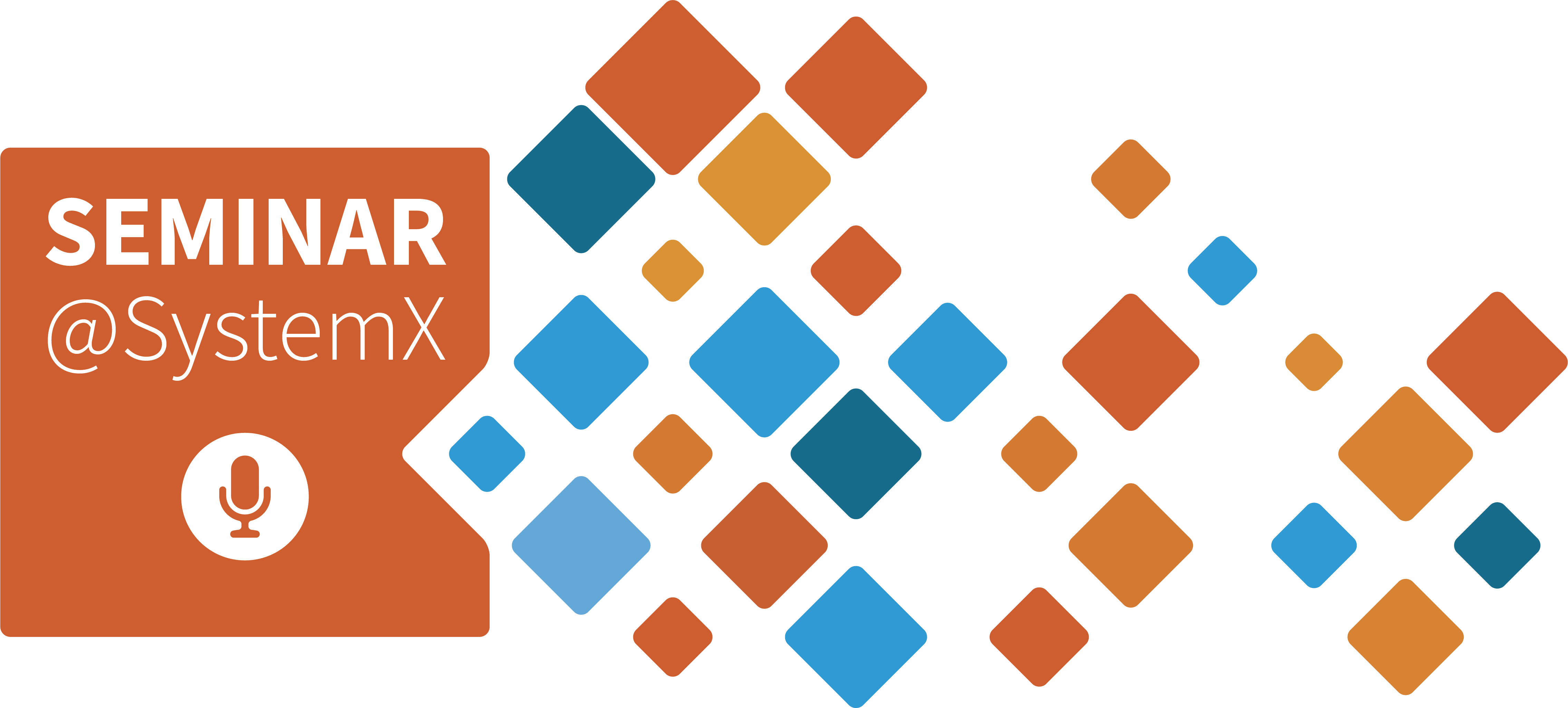
Seminar@SystemX animé par Pierre Colombo
Résume Biographie Inscription Pierre Colombo (CentraleSupélec - Equall.ai) animera un Seminar@SystemX sur le thème "Advancing ... En savoir plus
![[Save the Date] Workshop Cockpit Studio Lab](https://www.irt-systemx.fr/wp-content/uploads/2024/07/Capture-decran-2024-07-08-a-16.29.40.png)
[Save the Date] Workshop Cockpit Studio Lab
Explorez les défis de la conduite de demain et découvrez Cockpit Studio Lab, la plateforme de simulation et son cockpit immersif. En savoir plus
Nos dernières actualités
SystemX et LINAGORA collaborent pour renforcer la confiance dans les LLMs
L’IRT SystemX annoncent la...
Compétition ML4CFD NeurIPS 2024 : « Harnessing Machine Learning for Computational Fluid Dynamics in Airfoil Design »
Vous êtes expert en...
Cyber for Industry Day : le rendez-vous incontournable de la cybersécurité pour l’industrie
Le 20 juin, près de 100...